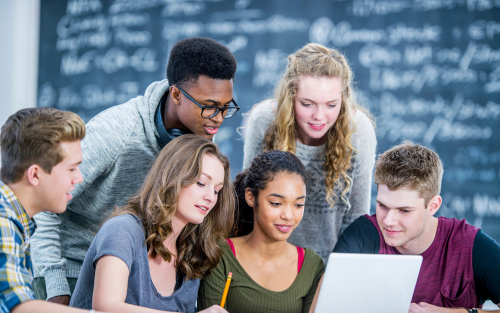
15 Feb How Can ChatGPT Be Used To Improve The Accuracy Of Recommendation Engines?

Recommendation engines have become an essential part of many businesses, especially in the e-commerce industry. These engines provide personalized product recommendations to customers based on their browsing and purchase history, preferences, and behavior. One of the challenges in developing a recommendation engine is improving its accuracy, which can significantly impact customer satisfaction and revenue. Artificial intelligence (AI) and natural language processing (NLP) technology, such as ChatGPT, can be used to improve the accuracy of recommendation engines. In this article, we will explore how ChatGPT can be used to improve recommendation engine accuracy.
What is ChatGPT?
ChatGPT is a large language model developed by OpenAI. It is based on the GPT (Generative Pre-trained Transformer) architecture and uses machine learning algorithms to generate text that is similar to human writing. ChatGPT has been trained on a massive amount of data and can produce high-quality content that is grammatically correct, coherent, and contextually relevant.
ChatGPT has many applications in natural language processing, including language translation, chatbots, and content generation. However, one of its most promising applications is in improving the accuracy of recommendation engines.
How Can ChatGPT Improve Recommendation Engine Accuracy?
ChatGPT can improve recommendation engine accuracy in several ways. Here are a few examples:
Understanding Customer Feedback
Recommendation engines rely on customer feedback to improve their accuracy. This feedback can come in many forms, such as ratings, reviews, and comments. However, processing and analyzing this feedback can be challenging, especially when it comes to understanding the nuances of human language.
ChatGPT can be used to process and analyze customer feedback more accurately. It can analyze text-based feedback, such as reviews and comments, and extract meaningful insights from them. For example, it can identify common themes and sentiments in customer feedback and use them to improve recommendation accuracy.
Generating Product Descriptions
Product descriptions play a crucial role in recommendation engines. They provide information about products that can help engines recommend the most relevant products to customers. However, creating accurate and engaging product descriptions can be time-consuming and challenging.
ChatGPT can be used to generate product descriptions automatically. It can analyze product data and generate descriptions that are accurate, informative, and engaging. This can help recommendation engines provide more relevant product recommendations to customers.
Improving Natural Language Processing
Natural language processing (NLP) is essential in recommendation engines. NLP algorithms analyze customer data, such as search queries and product descriptions, to identify patterns and make accurate recommendations. However, NLP algorithms can struggle with understanding the nuances of human language.
ChatGPT can improve NLP algorithms by providing more accurate and contextually relevant language processing. It can identify the underlying meaning of customer data and provide more accurate recommendations based on that understanding.
Generating Personalized Recommendations
Personalized recommendations are the cornerstone of effective recommendation engines. Personalization involves analyzing customer data, such as browsing and purchase history, to provide tailored recommendations that meet their needs and preferences. However, creating accurate and personalized recommendations can be challenging, especially when dealing with large amounts of data.
ChatGPT can be used to generate personalized recommendations automatically. It can analyze customer data and generate recommendations that are tailored to their preferences and behavior. This can help recommendation engines provide more accurate and relevant recommendations to customers.
How Can Businesses Implement ChatGPT in Recommendation Engines?
Implementing ChatGPT in recommendation engines requires a multi-step approach. Here are some steps businesses can take:
Define the Problem: The first step in implementing ChatGPT in recommendation engines is to define the problem. This involves identifying the areas where recommendation engines can be improved and determining how ChatGPT can help.
Gather Data: The next step in implementing ChatGPT in recommendation engines is to gather data. Data is the foundation of recommendation engines, and the quality of the data directly impacts the accuracy of the recommendations. There are several types of data that businesses need to gather, including:
Customer data: This includes data about customers’ browsing and purchase history, demographics, preferences, and behavior. This data is critical in creating personalized recommendations.
Product data: This includes data about products, such as descriptions, attributes, and ratings. This data is essential in creating accurate recommendations.
Feedback data: This includes data from customer feedback, such as reviews, ratings, and comments. This data can provide valuable insights into customer preferences and behavior.
Gathering data can be a challenging and time-consuming process. However, there are several ways businesses can gather data, including:
Web scraping: Web scraping involves extracting data from websites and online sources. This can be an effective way to gather product data and feedback data.
Surveys: Surveys can be used to gather customer data and feedback data. Businesses can use online survey tools to create and distribute surveys to customers.
Data partnerships: Businesses can partner with data providers to access high-quality data. This can be an effective way to gather customer and product data.
Once businesses have gathered data, they need to clean and preprocess it. Data cleaning involves removing duplicates, errors, and inconsistencies in the data. Preprocessing involves transforming the data into a format that can be used by recommendation engines, such as converting text data into numerical representations.
Train ChatGPT
The next step in implementing ChatGPT in recommendation engines is to train the model. This involves feeding the model with large amounts of data and fine-tuning it to improve its accuracy in specific tasks. Businesses can use existing pre-trained ChatGPT models and fine-tune them for recommendation engine tasks.
Training ChatGPT involves the following steps:
Data preparation: Businesses need to prepare the data for training. This involves splitting the data into training, validation, and testing sets.
Fine-tuning the model: Fine-tuning involves adjusting the model’s parameters to improve its accuracy in recommendation engine tasks. Businesses can use transfer learning techniques to fine-tune existing pre-trained ChatGPT models.
Evaluation: Businesses need to evaluate the performance of the fine-tuned ChatGPT model. This involves testing the model on the validation and testing sets to determine its accuracy.
Integrate ChatGPT into Recommendation Engine
The final step in implementing ChatGPT in recommendation engines is to integrate the model into the engine. This involves using the fine-tuned ChatGPT model to process customer data and generate personalized recommendations.
Integrating ChatGPT into recommendation engines involves the following steps:
Preprocessing customer data: Businesses need to preprocess customer data, such as search queries and browsing history, to prepare it for ChatGPT processing.
Processing customer data with ChatGPT: ChatGPT is used to process the preprocessed customer data and generate personalized recommendations.
Integrating recommendations into the engine: The personalized recommendations generated by ChatGPT are integrated into the recommendation engine and presented to customers.
Conclusion
In conclusion, ChatGPT can be used to improve the accuracy of recommendation engines. It can be used to process and analyze customer feedback, generate product descriptions, improve natural language processing, and generate personalized recommendations. Implementing ChatGPT in recommendation engines requires a multi-step approach, including defining the problem, gathering data, training ChatGPT, and integrating it into the recommendation engine. By using ChatGPT, businesses can improve the accuracy of their recommendation engines, provide more relevant recommendations to customers, and increase customer satisfaction and revenue.
Latest Topic
-
Cloud-Native Technologies: Best Practices
20 April, 2024 -
Generative AI with Llama 3: Shaping the Future
15 April, 2024 -
Mastering Llama 3: The Ultimate Guide
10 April, 2024
Category
- Assignment Help
- Homework Help
- Programming
- Trending Topics
- C Programming Assignment Help
- Art, Interactive, And Robotics
- Networked Operating Systems Programming
- Knowledge Representation & Reasoning Assignment Help
- Digital Systems Assignment Help
- Computer Design Assignment Help
- Artificial Life And Digital Evolution
- Coding and Fundamentals: Working With Collections
- UML Online Assignment Help
- Prolog Online Assignment Help
- Natural Language Processing Assignment Help
- Julia Assignment Help
- Golang Assignment Help
- Design Implementation Of Network Protocols
- Computer Architecture Assignment Help
- Object-Oriented Languages And Environments
- Coding Early Object and Algorithms: Java Coding Fundamentals
- Deep Learning In Healthcare Assignment Help
- Geometric Deep Learning Assignment Help
- Models Of Computation Assignment Help
- Systems Performance And Concurrent Computing
- Advanced Security Assignment Help
- Typescript Assignment Help
- Computational Media Assignment Help
- Design And Analysis Of Algorithms
- Geometric Modelling Assignment Help
- JavaScript Assignment Help
- MySQL Online Assignment Help
- Programming Practicum Assignment Help
- Public Policy, Legal, And Ethical Issues In Computing, Privacy, And Security
- Computer Vision
- Advanced Complexity Theory Assignment Help
- Big Data Mining Assignment Help
- Parallel Computing And Distributed Computing
- Law And Computer Science Assignment Help
- Engineering Distributed Objects For Cloud Computing
- Building Secure Computer Systems Assignment Help
- Ada Assignment Help
- R Programming Assignment Help
- Oracle Online Assignment Help
- Languages And Automata Assignment Help
- Haskell Assignment Help
- Economics And Computation Assignment Help
- ActionScript Assignment Help
- Audio Programming Assignment Help
- Bash Assignment Help
- Computer Graphics Assignment Help
- Groovy Assignment Help
- Kotlin Assignment Help
- Object Oriented Languages And Environments
- COBOL ASSIGNMENT HELP
- Bayesian Statistical Probabilistic Programming
- Computer Network Assignment Help
- Django Assignment Help
- Lambda Calculus Assignment Help
- Operating System Assignment Help
- Computational Learning Theory
- Delphi Assignment Help
- Concurrent Algorithms And Data Structures Assignment Help
- Machine Learning Assignment Help
- Human Computer Interface Assignment Help
- Foundations Of Data Networking Assignment Help
- Continuous Mathematics Assignment Help
- Compiler Assignment Help
- Computational Biology Assignment Help
- PostgreSQL Online Assignment Help
- Lua Assignment Help
- Human Computer Interaction Assignment Help
- Ethics And Responsible Innovation Assignment Help
- Communication And Ethical Issues In Computing
- Computer Science
- Combinatorial Optimisation Assignment Help
- Ethical Computing In Practice
- HTML Homework Assignment Help
- Linear Algebra Assignment Help
- Perl Assignment Help
- Artificial Intelligence Assignment Help
- Uncategorized
- Ethics And Professionalism Assignment Help
- Human Augmentics Assignment Help
- Linux Assignment Help
- PHP Assignment Help
- Assembly Language Assignment Help
- Dart Assignment Help
- Complete Python Bootcamp From Zero To Hero In Python Corrected Version
- Swift Assignment Help
- Computational Complexity Assignment Help
- Probability And Computing Assignment Help
- MATLAB Programming For Engineers
- Introduction To Statistical Learning
- Database Systems Implementation Assignment Help
- Computational Game Theory Assignment Help
- Database Assignment Help
- Probabilistic Model Checking Assignment Help
- Mathematics For Computer Science And Philosophy
- Introduction To Formal Proof Assignment Help
- Creative Coding Assignment Help
- Foundations Of Self-Programming Agents Assignment Help
- Machine Organization Assignment Help
- Software Design Assignment Help
- Data Communication And Networking Assignment Help
- Computational Biology
- Data Structure Assignment Help
- Foundations Of Software Engineering Assignment Help
- Mathematical Foundations Of Computing
- Principles Of Programming Languages Assignment Help
- Software Engineering Capstone Assignment Help
- Algorithms and Data Structures Assignment Help
No Comments