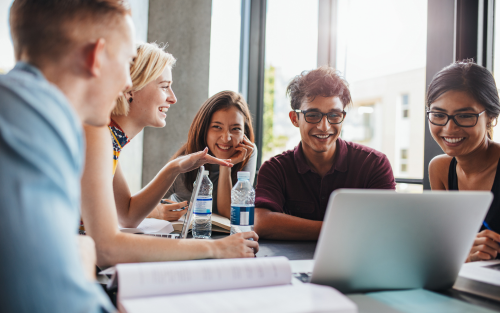
05 May MATLAB For Time Series Analysis And Forecasting

Introduction
Time series analysis and forecasting involve analyzing and modeling time-dependent data to make predictions about future trends. MATLAB is a powerful tool for time series analysis and forecasting due to its vast array of built-in functions and toolboxes. In this article, we will explore the benefits of using MATLAB for time series analysis and forecasting, as well as some of its applications.
Unlock the potential of MATLAB for time series analysis and forecasting. MATLAB offers a comprehensive suite of tools and functions specifically designed for analyzing and predicting time series data. With MATLAB, you can perform advanced statistical analysis, apply various forecasting models, and visualize time series data for better insights. Leverage MATLAB’s powerful computational capabilities to uncover patterns, trends, and correlations in your time series data and make accurate predictions for future trends. MATLAB simplifies the process of time series analysis and forecasting, enabling you to make informed decisions and optimize your business strategies.
Benefits of Using MATLAB for Time Series Analysis and Forecasting
Easy Data Import and Preprocessing: MATLAB provides easy data import and preprocessing tools for time series data, including built-in functions for reading data from various file formats and data sources, and data visualization tools for data exploration.
Extensive Time Series Analysis and Forecasting Toolboxes: MATLAB provides a variety of toolboxes for time series analysis and forecasting, including the Econometrics Toolbox, the Statistics and Machine Learning Toolbox, and the Neural Network Toolbox. These toolboxes provide a comprehensive set of functions for time series analysis and forecasting, including autoregressive integrated moving average (ARIMA) models, exponential smoothing models, seasonal decomposition, spectral analysis, and more.
Efficient Data Handling: MATLAB’s matrix-based data handling capabilities make it easy to manipulate large datasets, which is important for time series analysis and forecasting. MATLAB’s parallel computing capabilities can also be used to speed up computationally intensive time series analysis and forecasting tasks.
Advanced Visualization Tools: MATLAB provides advanced visualization tools for time series data, including interactive plots, histograms, and 3D plots. These visualization tools make it easy to explore and analyze time series data, and to communicate results effectively.
Integration with Other Tools: MATLAB can be integrated with other tools and languages, such as Python and R, which expands the range of functions available for time series analysis and forecasting. This integration also makes it easy to incorporate MATLAB code into larger projects that use other programming languages.
Applications of MATLAB in Time Series Analysis and Forecasting
MATLAB is widely used for time series analysis and forecasting due to its built-in functionalities and easy-to-use interface. Here are some of the common applications of MATLAB in this field:
Time Series Data Visualization: MATLAB provides various tools and functions for visualizing time series data. These include plot, stem, stairs, and bar functions, among others. Users can create various types of plots, such as line plots, scatter plots, and histogram plots, to visualize the patterns and trends in the data.
Time Series Analysis: MATLAB has several built-in functions for analyzing time series data. These include autocorrelation and cross-correlation functions, power spectral density estimation, and time-frequency analysis functions. Users can use these functions to identify the patterns, trends, and seasonal components in the data, as well as to detect any anomalies or outliers.
Time Series Modeling: MATLAB has several built-in functions for modeling time series data. These include autoregressive (AR) models, moving average (MA) models, and autoregressive integrated moving average (ARIMA) models. Users can use these models to create forecasts and predictions based on historical data.
Machine Learning: MATLAB has several machine learning functions and toolboxes that can be used for time series analysis and forecasting. These include support vector machines (SVM), neural networks, and decision trees, among others. Users can use these techniques to create more accurate and complex models for forecasting.
Forecasting: MATLAB provides several functions for forecasting future values of a time series based on historical data. These include exponential smoothing, ARIMA models, and neural network models, among others. Users can use these functions to create forecasts and predictions of future values of a time series based on historical data.
Signal Processing: MATLAB has several built-in signal processing functions that can be used for time series analysis and forecasting. These include filtering functions, such as low-pass, high-pass, and band-pass filters, as well as transform functions, such as Fourier transform and wavelet transform. These functions can be used to preprocess the data and extract useful features for modeling and forecasting.
Optimization: MATLAB has several optimization functions that can be used for time series analysis and forecasting. These include nonlinear optimization, linear programming, and constrained optimization functions, among others. Users can use these functions to optimize the parameters of the models and improve the accuracy of the forecasts.
Overall, MATLAB provides a comprehensive set of tools and functions for time series analysis and forecasting. Users can easily preprocess, analyze, model, and forecast time series data using MATLAB, making it an essential tool for researchers and practitioners in this field.
FAQs
What is time series analysis, and how is MATLAB used for it?
Time series analysis involves analyzing and modeling data that is collected sequentially over time. MATLAB provides a comprehensive set of functions and toolboxes specifically designed for time series analysis tasks.
Can MATLAB handle large-scale time series data?
Yes, MATLAB has efficient data handling capabilities, and it can handle large-scale time series data efficiently using techniques such as memory management, parallel computing, and data streaming.
What types of time series analysis techniques are available in MATLAB?
MATLAB offers various techniques for time series analysis, including trend analysis, seasonal decomposition, autocorrelation analysis, spectral analysis, ARIMA modeling, and machine learning-based forecasting models.
Can MATLAB perform advanced statistical analysis on time series data?
Yes, MATLAB provides a range of statistical functions and toolboxes that enable advanced analysis of time series data, including hypothesis testing, parameter estimation, model diagnostics, and outlier detection.
How can MATLAB be used for time series forecasting?
MATLAB offers powerful forecasting capabilities, allowing users to build and evaluate forecasting models, including ARIMA, exponential smoothing, neural networks, and machine learning-based models, to predict future values of a time series.
Is MATLAB suitable for real-time time series analysis and forecasting?
Yes, MATLAB supports real-time time series analysis and forecasting by providing optimized algorithms and efficient data processing techniques, making it suitable for applications that require real-time decision-making.
Can MATLAB handle multivariate time series analysis?
Yes, MATLAB supports multivariate time series analysis, allowing users to analyze and model relationships between multiple variables over time using techniques such as vector autoregression (VAR) and dynamic factor models.
Are there any specialized toolboxes or functions in MATLAB for time series analysis and forecasting?
Yes, MATLAB offers specialized toolboxes like the Econometrics Toolbox and the Statistics and Machine Learning Toolbox, which provide dedicated functions and algorithms for time series analysis and forecasting tasks.
Can MATLAB visualize and plot time series data?
Yes, MATLAB has extensive visualization capabilities, allowing users to create informative plots, time series graphs, trend plots, seasonal plots, and forecasting plots to visually analyze and interpret time series data.
Are there any limitations or considerations to keep in mind when using MATLAB for time series analysis and forecasting?
While MATLAB is a powerful tool for time series analysis and forecasting, it is essential to consider factors such as data quality, model assumptions, and validation techniques to ensure accurate and reliable results. Additionally, handling large-scale time series data may require appropriate computational resources and optimization techniques.
Conclusion
MATLAB provides a wide range of built-in functions and tools for time series analysis and forecasting, including signal processing, spectral analysis, and regression analysis. Its ability to handle large datasets, perform data preprocessing and cleaning, and generate accurate predictions and forecasts makes it a valuable tool for researchers, analysts, and decision-makers.
The availability of numerous toolboxes, such as the Statistics and Machine Learning Toolbox and the Econometrics Toolbox, enables users to perform a variety of advanced time series analysis and forecasting tasks, such as spectral density estimation, wavelet analysis, and nonlinear time series modeling. The ability to integrate external data sources, such as economic indicators or climate data, further enhances the capabilities of MATLAB in time series analysis and forecasting.
In summary, the use of MATLAB in time series analysis and forecasting offers numerous benefits, including its ability to handle large datasets, perform data preprocessing and cleaning, generate accurate predictions and forecasts, and provide a user-friendly interface. Its flexibility and versatility make it a valuable tool for researchers, analysts, and decision-makers in a wide range of fields.
Latest Topic
-
Cloud-Native Technologies: Best Practices
20 April, 2024 -
Generative AI with Llama 3: Shaping the Future
15 April, 2024 -
Mastering Llama 3: The Ultimate Guide
10 April, 2024
Category
- Assignment Help
- Homework Help
- Programming
- Trending Topics
- C Programming Assignment Help
- Art, Interactive, And Robotics
- Networked Operating Systems Programming
- Knowledge Representation & Reasoning Assignment Help
- Digital Systems Assignment Help
- Computer Design Assignment Help
- Artificial Life And Digital Evolution
- Coding and Fundamentals: Working With Collections
- UML Online Assignment Help
- Prolog Online Assignment Help
- Natural Language Processing Assignment Help
- Julia Assignment Help
- Golang Assignment Help
- Design Implementation Of Network Protocols
- Computer Architecture Assignment Help
- Object-Oriented Languages And Environments
- Coding Early Object and Algorithms: Java Coding Fundamentals
- Deep Learning In Healthcare Assignment Help
- Geometric Deep Learning Assignment Help
- Models Of Computation Assignment Help
- Systems Performance And Concurrent Computing
- Advanced Security Assignment Help
- Typescript Assignment Help
- Computational Media Assignment Help
- Design And Analysis Of Algorithms
- Geometric Modelling Assignment Help
- JavaScript Assignment Help
- MySQL Online Assignment Help
- Programming Practicum Assignment Help
- Public Policy, Legal, And Ethical Issues In Computing, Privacy, And Security
- Computer Vision
- Advanced Complexity Theory Assignment Help
- Big Data Mining Assignment Help
- Parallel Computing And Distributed Computing
- Law And Computer Science Assignment Help
- Engineering Distributed Objects For Cloud Computing
- Building Secure Computer Systems Assignment Help
- Ada Assignment Help
- R Programming Assignment Help
- Oracle Online Assignment Help
- Languages And Automata Assignment Help
- Haskell Assignment Help
- Economics And Computation Assignment Help
- ActionScript Assignment Help
- Audio Programming Assignment Help
- Bash Assignment Help
- Computer Graphics Assignment Help
- Groovy Assignment Help
- Kotlin Assignment Help
- Object Oriented Languages And Environments
- COBOL ASSIGNMENT HELP
- Bayesian Statistical Probabilistic Programming
- Computer Network Assignment Help
- Django Assignment Help
- Lambda Calculus Assignment Help
- Operating System Assignment Help
- Computational Learning Theory
- Delphi Assignment Help
- Concurrent Algorithms And Data Structures Assignment Help
- Machine Learning Assignment Help
- Human Computer Interface Assignment Help
- Foundations Of Data Networking Assignment Help
- Continuous Mathematics Assignment Help
- Compiler Assignment Help
- Computational Biology Assignment Help
- PostgreSQL Online Assignment Help
- Lua Assignment Help
- Human Computer Interaction Assignment Help
- Ethics And Responsible Innovation Assignment Help
- Communication And Ethical Issues In Computing
- Computer Science
- Combinatorial Optimisation Assignment Help
- Ethical Computing In Practice
- HTML Homework Assignment Help
- Linear Algebra Assignment Help
- Perl Assignment Help
- Artificial Intelligence Assignment Help
- Uncategorized
- Ethics And Professionalism Assignment Help
- Human Augmentics Assignment Help
- Linux Assignment Help
- PHP Assignment Help
- Assembly Language Assignment Help
- Dart Assignment Help
- Complete Python Bootcamp From Zero To Hero In Python Corrected Version
- Swift Assignment Help
- Computational Complexity Assignment Help
- Probability And Computing Assignment Help
- MATLAB Programming For Engineers
- Introduction To Statistical Learning
- Database Systems Implementation Assignment Help
- Computational Game Theory Assignment Help
- Database Assignment Help
- Probabilistic Model Checking Assignment Help
- Mathematics For Computer Science And Philosophy
- Introduction To Formal Proof Assignment Help
- Creative Coding Assignment Help
- Foundations Of Self-Programming Agents Assignment Help
- Machine Organization Assignment Help
- Software Design Assignment Help
- Data Communication And Networking Assignment Help
- Computational Biology
- Data Structure Assignment Help
- Foundations Of Software Engineering Assignment Help
- Mathematical Foundations Of Computing
- Principles Of Programming Languages Assignment Help
- Software Engineering Capstone Assignment Help
- Algorithms and Data Structures Assignment Help
No Comments